The Flexible Renewable Energy Materials and Devices (La FREMD) team led by researcher Sun Kuan from the School of Energy and Power Engineering of Chongqing University has cooperated with multiple research groups inside and outside the university. Recently, it successfully realized the screening of organic photovoltaic materials through machine learning. "Machine learning assisted molecular design and efficiency prediction for high performance organic photovoltaic materials" is the topic, with Chongqing University as the first and communication unit in the Science sub-Journal "Science Advances" ( Impact factor = 12.8). Researcher Sun Kuan of Chongqing University, Researcher Lu Shirong and Researcher Xiao Zeyun of Chongqing Institute of Green and Intelligent Technology of Chinese Academy of Sciences are the co-corresponding authors. Master students Sun Wenbo, Dr. Zheng Yujie and doctoral student Yang Ke are co-first authors. So far, since Prof. Sun Kuan joined Chongqing University for 5 years, he has published 4 original papers as the first or corresponding author in the sub-journals of Cell, Nature and Science (CNS).
Organic solar cells are a direct and economical way to convert solar energy into electrical energy. In recent years, organic solar cell research has experienced rapid development, and the photoelectric conversion efficiency (PCE) has exceeded 17%. Until now, organic photovoltaic research has focused on establishing the relationship between the molecular structure of new materials and their photovoltaic properties. This process usually involves the design and synthesis of photovoltaic materials, the characterization of the photoelectric properties of the materials, and the assembly and optimization of photovoltaic cells. This traditional method includes fine control and optimization of chemical synthesis and device preparation, and requires a large amount of resource input and a long research period. Therefore, the development speed of organic photovoltaic materials has been slow. Since organic solar cells were first reported in 1973, fewer than 2,000 organic solar cell donor material molecules have been synthesized and tested in photovoltaic devices. However, the experimental data generated during this half-century of exploration is precious. However, so far, the potential value of data has not been fully utilized when searching for high-performance organic photovoltaic materials. In order to extract useful information from these data, a program that can scan a large number of data sets and extract the relationships between features is needed. Machine learning is an algorithm that meets the requirements. It provides a set of calculation tools that can learn and recognize patterns or relationships based on error (or loss function) minimization or probability rules (such as maximizing likelihood), and predict the results or make a decision. This data-driven approach enables machine learning to predict a wide range of material properties without the need for an in-depth understanding of the chemical or physical principles behind these properties.
Recently, the La FREMD team of the School of Energy and Power Engineering of Chongqing University has joined forces with the School of Automation, School of Computer, School of Economics and Management, North China University of Technology, and the Chongqing Institute of Green and Intelligent Technology of the Chinese Academy of Sciences to develop high-performance organic solar cell donor materials. In the process, machine learning is used to establish the relationship between chemical structure and photovoltaic characteristics before synthesizing new materials, and to predict the efficiency of new materials. The project first established a database containing more than 1,700 organic solar cell donor materials that have been reported in the literature. Through supervised learning, the machine learning model designed by the project can establish a "structure-performance" relationship, so as to achieve rapid screening of organic photovoltaic materials. The project explored several expressions of molecular structure, such as molecular structure diagrams, ASCII code strings, molecular descriptors, and molecular fingerprints as input to various machine learning algorithms. It was found that molecular fingerprints with a length of more than 1000 bits can obtain high prediction accuracy. Rate is the best expression for this type of machine learning. In addition, the author used the machine learning model to predict 10 newly designed donor materials, and the prediction results of the model and the experimental results have good consistency, thereby further verifying the reliability of the machine learning method. The results show that machine learning is a powerful tool for pre-evaluating and screening new organic photovoltaic materials, and this method can accelerate the development of organic solar cells.
The research work was funded by the National Natural Science Foundation of China, the National Instruments Special Project, the Hundred Talents Program of the Chinese Academy of Sciences, and the Chongqing Science and Technology Commission. The author also thanks Academician Yongfang Li of the Institute of Chemistry of the Chinese Academy of Sciences for his valuable comments on the research work.
Full text link:https://advances.sciencemag.org/content/5/11/eaay4275
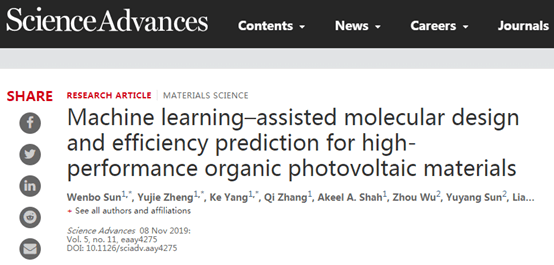